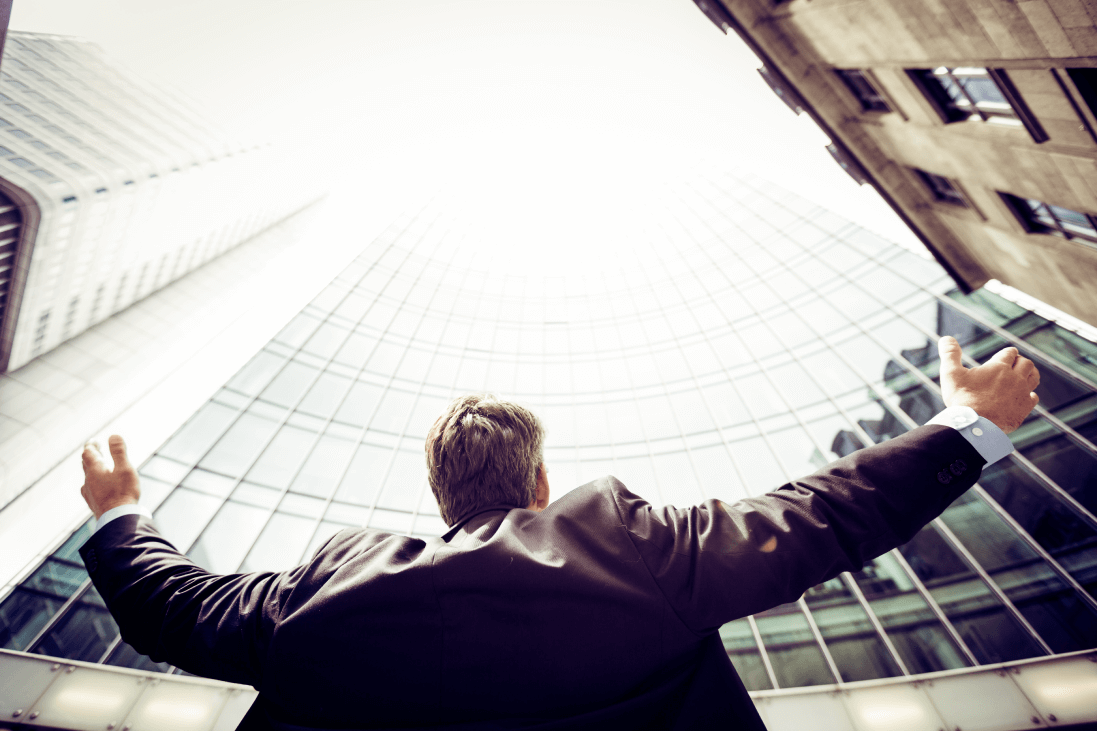
In a world of continuous product discovery and market exploration, businesses are doing experiments as they allow them to test and validate ideas at the quickest. And when it comes to choosing which experiments to run, human nature wins the day — the decisions are usually based on people’s inner confidence in being right in their assumptions.
But this approach doesn’t work in practice. What really works is making decisions based on something real like test results.
That is what I explained to a client at the workshop I was running earlier this year. The team was describing the work they planned to do, and we were defining the major customer problems to be solved and how solving those problems would deliver value to the company.
And one of the "things" they planned to make seemed wrong to me when I heard it for the first time. I asked the team how confident they were that delivering this thing would lead to the desired outcomes.
Their answer was "80% confident".
Then I shared the score-based scale of confidence (see below), which I developed as part of the LeadingAgile team while working with another client. The scale ranges from "no reason at all to be confident" to "very good reason to be confident." Higher numbers reflect a greater likelihood that the assumption is correct.
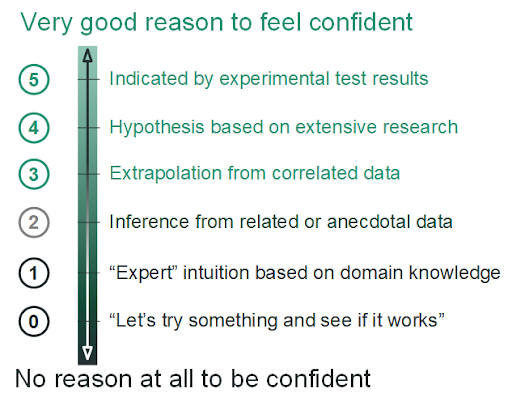
I asked the team to re-assess their confidence according to the scale.
That caused some chuckles and groans, and a chorus of "zero" and "one" outbursts filled the room.
That’s how the client understood that they should base their decisions on how they are justified in their confidence, not on their assumptions or intuition.
Confidence scale in impact mapping
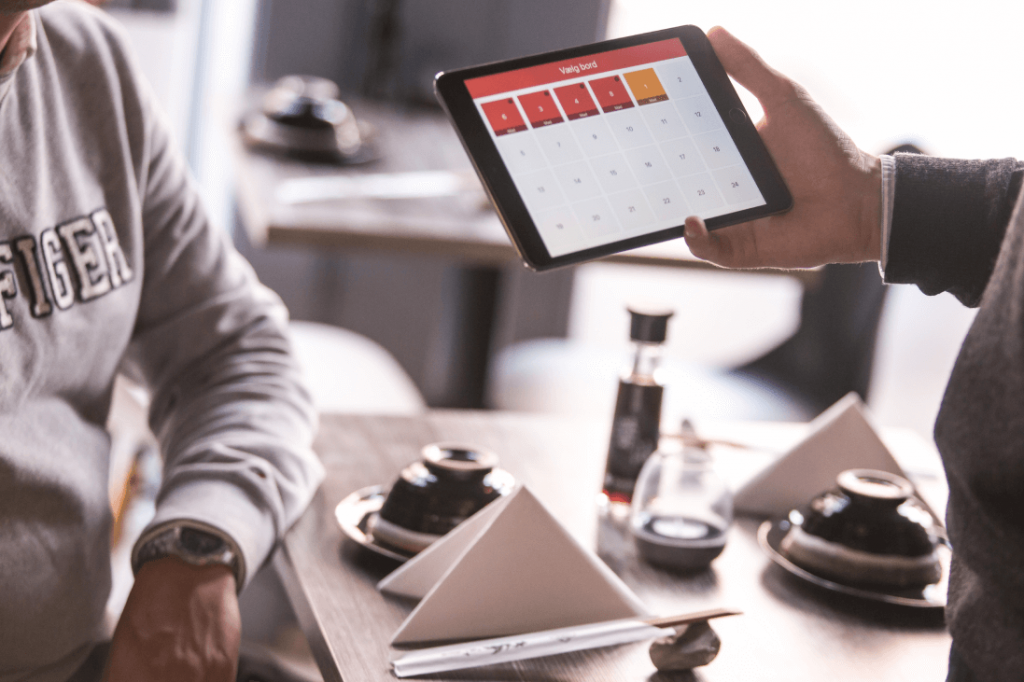
A collection of hypotheses is one way to interpret empathy maps. These can be problem-solution and outcome hypotheses.
Problem-solution hypotheses look as follows: if we make this thing, then it will enable or cause a change in behavior (because it solves a specific problem).
Outcome hypotheses focus on business outcomes: if we enable or cause a change in the behavior of a particular actor, then it will result in a business outcome.
You can use my scale to score each of these hypotheses and make better decisions for your business.
Let’s imagine that you want to introduce a new feature to your product that will cause a behavior change. It can be profile personalization, for instance. Do some research and use the scale to come up with the statement:
with confidence justification of 4, we believe that adding the ability to personalize a profile
will increase the engagement, resulting in 5% of website visitors engaging with
the site 10% more frequently, based on the behavioral psychology research
citation below.
The hypothesis has a score of 4, which shows that the experiment based on it is highly likely to be successful. So put it high on the list of upcoming experiments.
For an outcome hypothesis, this could be the following statement:
with confidence
justification of 2, we believe a 10% increase in user retention will result in
a 10% increase in repeat sales, because repeat sales seem to have a steady
frequency, so being around longer equates to more purchases.
It means that the experiment based on the statement should be a lower priority than those based on the statements with higher confidence scores.
Using the confidence scale in journey maps
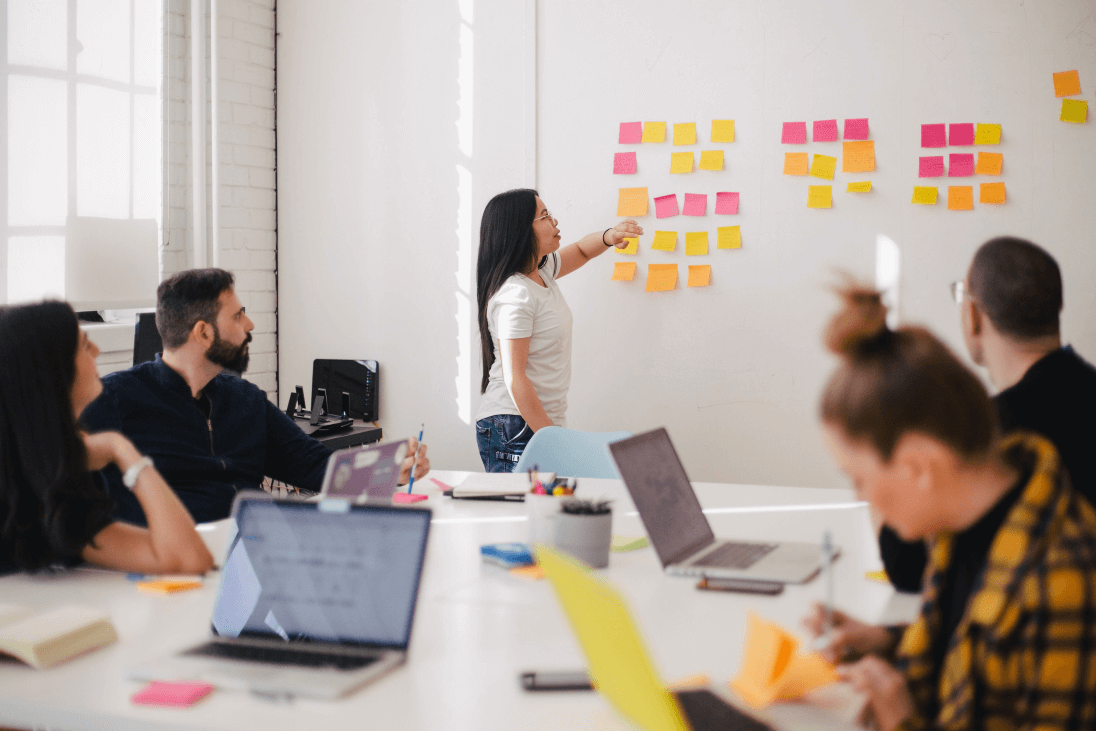
The scale of confidence can also be useful when building a customer journey map. One of the ways to create one is to identify the problems a user faces at each stage of the journey. And the scale of confidence can help you with that.
When choosing what to build next, you are assuming (a) that the thing you make will solve the problem, and (b) that solving this problem will improve the user's experience by some amount at the given stage, and (c) that this improvement will lead to the desired outcome that will benefit your business.
You can apply the scale to each of these hypotheses and prioritize your business experiments.
Wrap up
The experimental mindset is what drives innovation at companies these days. But choosing the “right” experiments to run can be hard, as innovation teams rely more on their intuition and assumptions than on real data.
The approaches I described allow you to identify the riskiest parts of the plan and de-risk it before you start an experiment.
About the author
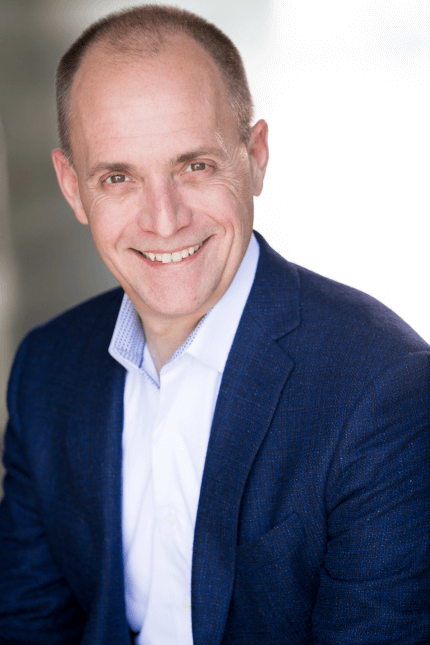
Scott Sehlhorst is a product management and strategy consultant. He continues as president of Tyner Blain since 2005, and is also a senior vice president and executive consultant at LeadingAgile, driving transformation at very large companies. Scott is also a visiting professor of product management at Lviv Business School.